12 ways Predictive Analytics is changing the E-commerce Industry- Part 1
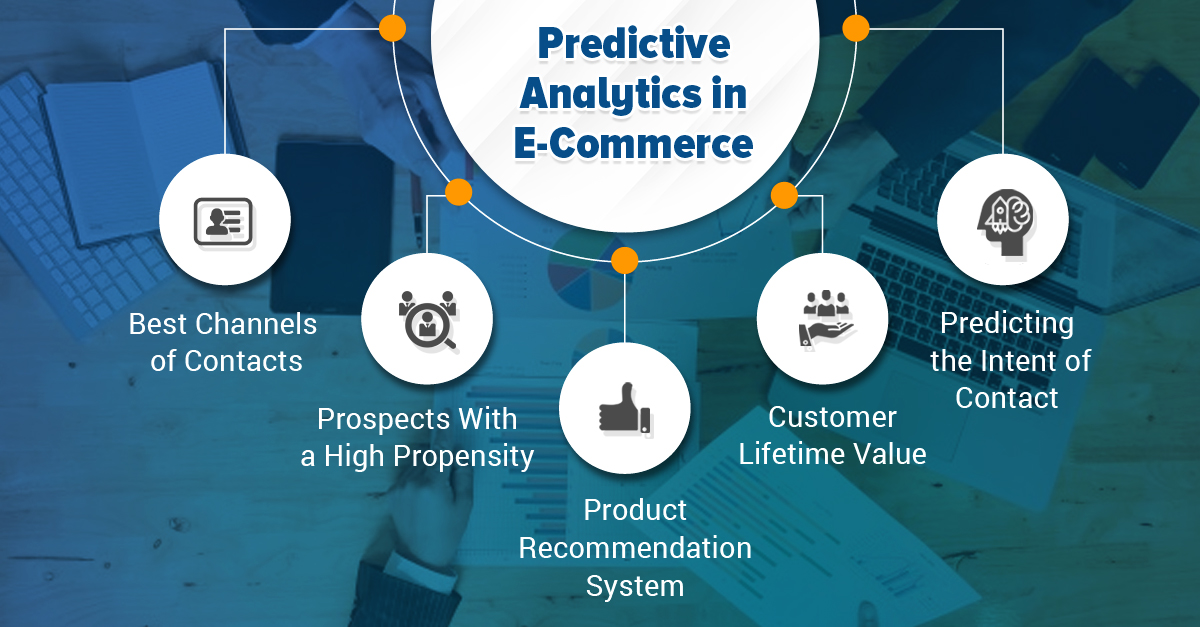
Predictive analytics has been around for decades, but its practical applications have only begun to reach the limelight.
Predictive analytics is used to extract information from existing data sets to make precise predictions of future events using the sciences of advanced analytics. The main techniques used are artificial intelligence are machine learning, data mining, statistical algorithms, modeling, machine learning, etc. The algorithms used will generate a forecast of what might occur in the future with a high degree of reliability.
The predictions may also include what-if scenario analysis [like Monte Carlo analysis] and risk assessment results.
Predictive analysis has been revolutionizing the e-commerce industry with its predictive customer analytics capabilities. Now, with the emergence of big data, affordable and easily implementable predictive analytics solutions are available in the market.
How is predictive analytics radically changing the face of e-commerce?
With the emergence of AI in e-commerce, predictive analytics is being used in a lot of domains.
There are a lot of agencies that provide e-commerce predictive analytics consulting services that cover most of the predictive analytics solutions for your company. Below are the six main applications of the same in the e-commerce industry:
- Finding the best channels of contacts
Predictive analytics can help you reach target prospects using the best channels possible. Demographics data like age, gender, income, family, location are collected along with past successful events like opened emails, clicked ads, unpurchased products in the cart, etc. This data is then fed into AI-based predictive algorithms to identify the best way to attract and retain the customers.
- Finding prospects with a high propensity
Propensity modeling is a well-researched topic under data science and analytics today. Many AI predictive analytics services are already giving propensity modeling as a service to find prospective buyers with buying chances. This model studies the features of the customer to calculate buying and non-buying probabilities. It helps to identify potential buyers who have a high likelihood of buying your products. Better deals can be offered to these prospects, thus increasing the probability of conversions.
- Predicting customer propensity
The initial papers on propensity modeling were published in 1983. Since then, this part of predictive analytics has grown. Propensity modeling is all about predicting whether visitors, leads, will perform specific actions or not. Customer propensity is determined by monitoring real-time actions being performed by the customer on the website/mobile app. This is basically done to extract information from customer engagement and use that information to sell products.
- Product recommendation system
A system that filters out products that current user is likely to buy is a big boon to the e-commerce industry. For the sellers, a product recommendation system is all that they need because all visitors are quickly going to be converted to sales. For buyers, they get to see what they need in the first screen itself, and they won’t have to dig deep into the website. A sound product recommendation system based on predictive analytics algorithms creates a win-win situation for buyers and sellers.
Recommendation systems also help to implement the marketing concepts of cross-selling and up-selling, thus increasing sales conversions.
- Predicting Customer Lifetime Value
Customer Lifetime Value (CLV) is the discounted value of future profits generated by the customer. By formula, Lifetime Value is calculated as Lifetime Value=Total Gross Revenue – Total Cost
Customers generate revenue for the company in different ways. Direct purchase is a source of direct income for the company. There may also be indirect revenue generated when the customer refers to your company products to his friends, and they make a purchase. Thus, at any point in time, customer CLV is analyzed by prediction algorithms using historic CLV trends. Using CLV, businesses can now better target those customers who can bring in more value in return.
- Predicting the intent of contact
A predictive analytics solution can identify the intent of a customer when he contacts a business.
This is one of the most complex parts of predictive analytics involving the customer life cycle, acquiring customer data. Analytics solutions may also test customer loyalty by throwing test cases at customers to discover and understand response patterns.
Another side to this is identifying dissatisfied customers and uncovering attrition patterns. The intent analysis helps to provide the right level of customer service to deserving customers, thereby increasing customer satisfaction. Intent prediction gives rise to another form of marketing called intent marketing, wherein the intent of customers is analyzed from all transactions.
Winding-up
There is a lot of value that predictive analytics can bring to businesses which are running on online platforms. Customers, too, can benefit from these predictions by scoring personalized offers and being ‘magically’ shown the product they were googling about a few days back.
All in all, the understanding between businesses and customers is growing because of predictive customer analytics and improving the overall business scenario. We’ll cover more ground in Part 2!