MLOps – The New Mantra for Businesses in the AI and ML Game!
Thanks to the rapid adoption of Artificial Intelligence (AI) and Machine Learning (ML) across industries, AI and ML have found a place in the common vocabulary. Almost every sector of the industry (healthcare, e-commerce, IoT, banking & finance, etc.) are leveraging AI and ML to streamline business operations and create innovative products/services.
So, when everyone in the industry is using AI and ML, what can you do differently to up your game?
The answer is MLOps or Machine Learning Operationalization.
What is MLOps?
In simple terms, MLOps is the Machine learning equivalent of DevOps. While DevOps helped optimize the production lifecycle of Big Data project, MLOps seeks to solve the problems associated with the implementation of ML in production.
By nature, Machine Learning Operationalization is a deeply collaborative process. It encourages communication between data scientists and the operations or production team to enhance automation, minimize waste, and ultimately produce more productive and consistent insights with ML.
According to a Gartner Analyst and Research Director, Erick Brethenoux, while many companies develop ML models, only 47% of those models go into production. Also, nearly 88% of AI initiatives in the corporate sector struggle to move past the test stage.
Every ML model faces a host of challenges during the four core stages of its lifecycle:
- ETL (Data pipelines)
- Algorithm training
- Inference
- Monitoring, Management, and Updates
These challenges primarily relate to automation and deployment; diagnostics; predictions; scalability issues; governance and regulatory compliance; collaboration between teams, and business use cases.
Since ML involves numerous intricacies and complexities, standard DevOps processes aren’t enough to address these challenges. Thus, arose the need for a more comprehensive solution for ML implementation – MLOps.
From DevOps to MLOps
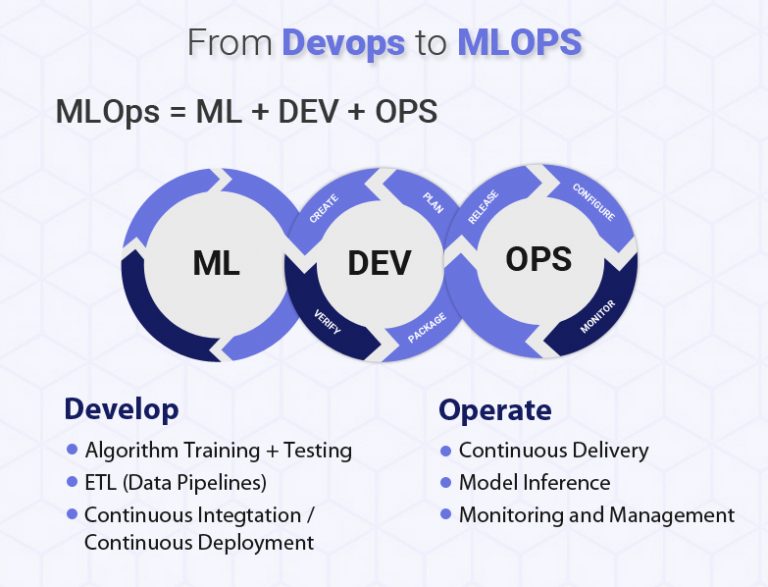
DevOps is an upgrade over the traditional approach. It is a software automation delivery process that facilitates the simultaneous functioning of software development and testing. Basically, DevOps automates the product development and delivery process by creating a collaborative working space for Software Developers and IT teams.
MLOps is founded on the very concept of DevOps (automation and collaboration between teams, but exclusively for AI/ML models. By leveraging the right AI/ML tools and frameworks, Machine Learning Operationalization seeks to establish a work environment that allows companies to boost their ROI. ML technologies can enable businesses to scale from development to testing to production quickly. Machine Learning Operationalization seamlessly links the development cycle of the ML model with its implementation cycle, thereby streamlining the entire process.
Why adopt MLOps?
For businesses implementing ML, MLOps should be a top priority. It can streamline business processes in the following ways:
- By allowing Data Scientists and Operations teams to work together, MLOps combines the power of business knowledge with data. This encourages data-driven business operations.
- MLOps can take care of regulatory and compliance issues. While it introduces the Operations team to the latest regulations and best industry practices, it allows the Data Science team to focus on building and deploying creative ML models.
- Machine Learning Operalization helps reduce the gap between discovering insights and turning those insights into actionable business plans.
To conclude, MLOps allows businesses to learn how to leverage data to take better control of production level ML. With MLOps, a business can implement cutting-edge ML practices to generate higher returns.